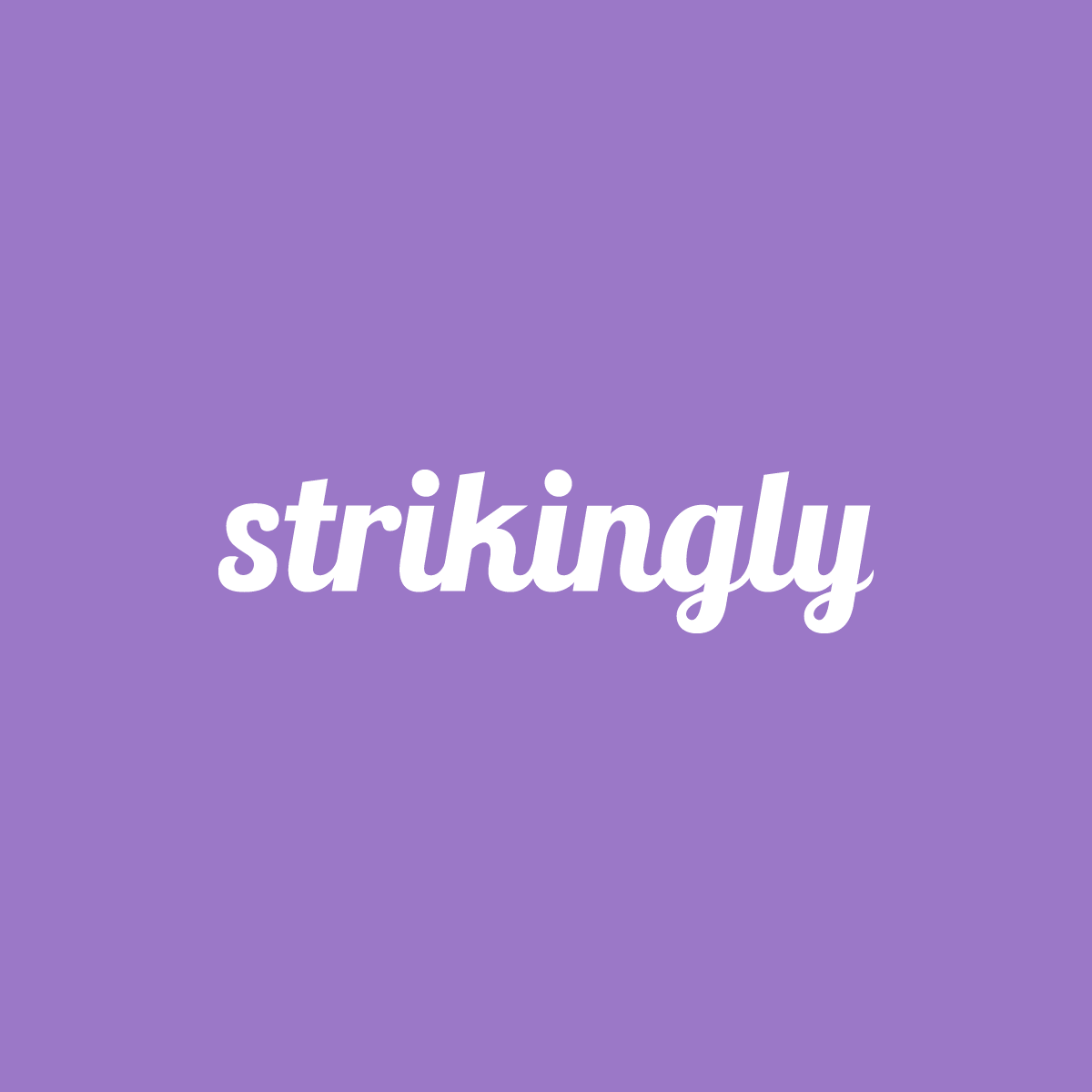
Machine Learninig
Machine Learning TrainingAI is a utilization of AI that empowers frameworks to gain and improve as a matter of fact without being expressly modified. AI centers around creating PC programs that can get to information and use it to find out on their own.
How Does Machine Learning Work?
Like how the human mind acquires information and understanding, AI depends on input, for example, preparing information or information charts, to grasp substances, areas and the associations between them. With elements characterized, profound learning can start.
The AI cycle starts with perceptions or information, like models, direct insight or guidance. It searches for designs in information so it can later make surmisings in view of the models gave. The essential point of ML is to permit PCs to advance independently without human intercession or help and change activities as needs be.
Why Is Machine Learning Important?
AI as an idea has been around for a long while. The expression "AI" was authored by Arthur Samuel, a PC researcher at IBM and a trailblazer in AI and PC gaming. Samuel planned a PC program for playing checkers. The more the program played, the more it gained as a matter of fact, utilizing calculations to make expectations.As a discipline, AI investigates the examination and development of calculations that can gain from and make expectations on information.
ML has demonstrated significant on the grounds that it can take care of issues at a speed and scale that can't be copied by the human brain alone. With gigantic measures of computational capacity behind a solitary assignment or different explicit errands, machines can be prepared to recognize designs in and connections between input information and computerize routine cycles.
Information Is Key: The calculations that drive AI are basic to progress. ML calculations assemble a numerical model in light of test information, known as "preparing information," to pursue expectations or choices without being expressly modified to do as such. This can uncover patterns inside information that data organizations can use to further develop independent direction, improve effectiveness and catch noteworthy information at scale.
Simulated intelligence Is the Goal: ML gives the establishment to AI frameworks that mechanize processes and tackle information based business issues independently. It empowers organizations to supplant or expand specific human capacities. Normal AI applications you might find in reality incorporate chatbots, self-driving vehicles and discourse acknowledgment.AI Is Widely Adopted
AI isn't sci-fi. It is as of now broadly utilized by organizations across all areas to propel advancement and increment process proficiency. In 2021, 41% of organizations sped up their rollout of AI because of the pandemic. These newbies are joining the 31% of organizations that as of now have AI underway or are effectively directing AI advancements.Information security: Machine learning models can recognize information security weaknesses before they can transform into breaks. By seeing previous encounters, AI models can foresee future high-risk exercises so hazards can be proactively relieved.
Finance: Banks, exchanging businesses, and fintech firms use AI calculations to mechanize exchanging and to offer monetary warning types of assistance to financial backers. Bank of America is utilizing a chatbot, Erica, to robotize client assistance.
Medical services: ML is utilized to break down enormous medical services informational collections to speed up the revelation of therapies and fixes, work on understanding results, and mechanize routine cycles to forestall human mistakes. For instance, IBM's Watson utilizes information mining to give doctors the information they can use to customize patient treatment.
Misrepresentation recognition: AI is being utilized in the monetary and banking area to independently investigate enormous quantities of exchanges to reveal fake actions progressively. Innovation benefits firm Capgemini claims that extortion discovery frameworks utilizing AI and examination limit misrepresentation examination time by 70% and further develop identification precision by 90%.
Retail: AI scientists and designers are utilizing ML calculations to foster AI proposal motors that give significant item thoughts in view of purchasers' past decisions, as well as authentic, geographic, and segment information.Preparing Methods for Machine Learning Differ
AI offers clear advantages for AI innovations. However, which AI approach is appropriate for your association? There are numerous ML preparing techniques to look over including:managed learning
solo learning
semi-managed learning
We should find out what each brings to the table.Administered Learning: More Control, Less Bias
Administered AI calculations apply what has been realized in the past to new information utilizing marked guides to anticipate future occasions. By dissecting a known preparation dataset, the learning calculation delivers a construed capacity to anticipate yield values. The framework can give focus to any new contribution after adequate preparation. It can likewise contrast its result and the right, expected result to track down mistakes and adjust the model as needs be.Unaided Learning: Speed and Scale
Unaided AI calculations are utilized when the data used to prepare is neither arranged nor named. Unaided learning concentrates on how frameworks can deduce a capacity to portray a concealed construction from unlabeled information. Never does the framework know the right result with conviction. All things considered, it draws deductions from datasets concerning what the result ought to be.Support Learning: Rewards Outcomes
Support AI calculations are a learning strategy that interfaces with its current circumstance by delivering activities and finding blunders or rewards. The most applicable qualities of support learning are experimentation search and deferred reward. This strategy permits machines and programming specialists to consequently decide the best way of behaving inside a particular setting to boost its presentation. Straightforward award input — known as the support signal — is expected for the specialist to realize which activity is ideal.AI Is Not Perfect
It is critical to figure out what AI should or shouldn't do. However valuable as it seems to be in computerizing the exchange of human knowledge with machines, it is a long way from an ideal answer for your information-related issues. Consider the accompanying deficiencies before you jump excessively profound into the ML pool:AI isn't situated in information. In opposition to mainstream thinking, AI can't accomplish human-level knowledge. Machines are driven by information, not human information. Subsequently, "insight" is directed by the volume of information you need to prepare it with.
AI models are challenging to prepare. 81% of information researchers concede that preparing AI with information is more troublesome than anticipated. It requires investment and assets to prepare machines. Enormous informational collections are expected to make information models, and the interaction includes physically pre-labeling and ordering informational indexes. This asset channel can make inertness and bottlenecks in propelling ML drives.
AI is inclined to information issues. 96% of organizations have encountered preparing related issues with information quality, information naming and building model certainty. Those preparing related issues are a key motivation behind why 78% of ML projects slow down before sending. This has made an uncommonly high edge for ML achievement.
AI is frequently one-sided. AI frameworks are known for working in a black box, meaning you have zero ability to see how the machine learns and decides. In this way, on the off chance that you recognize a case of predisposition, it is absolutely impossible to distinguish what caused it. Your main plan of action is to retrain the calculation with extra information, however, that is no assurance to determining the issue.The Future of Machine Learning: Hybrid AI
For its deficiencies as a whole, AI is as yet basic to the progress of AI. This achievement, in any case, will be dependent upon one more way to deal with AI that counters its shortcomings, similar to the "black box" issue that happens when machines learn solo. That approach is representative AI or a standard-based procedure for handling information. A representative methodology utilizes an information diagram, which is an open box, to characterize ideas and semantic connections.Together, ML and representative AI structure crossover AI, a methodology that assists AI with figuring out language, not simply information. With more knowledge into what was realized and why, this strong methodology is changing the way that information is utilized across the venture.
Could it be said that you are a complete fledgling or a Machine Learning proficient who is searching for progress in your vocation? Then, at that point, Machine Learning training in Chennai gives far-reaching preparation for this strong concept. You can acquire better experiences on ML through our inside and out course.
Visit our other site : Machine Learning Trainings
SIGN UP
We'll get in touch with you soon.
Created With Strikingly.com